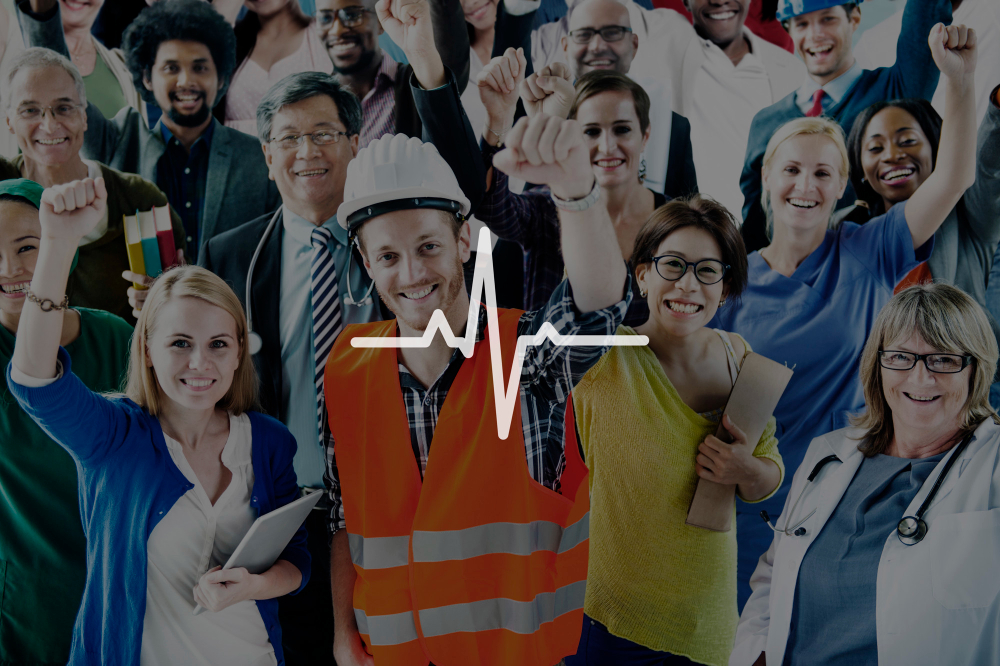
Population health management is a proactive approach to healthcare that focuses on improving a population’s overall health outcomes. It entails recognizing and resolving health problems on an individual and community level. In community health management, early disease detection and prevention are critical.
Predictive analytics uses modern technology and data analysis to identify those at high risk of getting specific diseases and allows healthcare practitioners to intervene early and execute preventative interventions by examining numerous aspects such as medical history, lifestyle, and genetic predispositions.
Understanding the Significance of Early Disease Detection and Prevention
The influence of early disease identification and prevention on population health outcomes is enormous. Traditional reactive healthcare systems have limitations since they focus on treating diseases after symptoms develop. They frequently result in delayed diagnosis and missing preventative chances. Proactive and predictive solutions, such as population health management, emphasize early identification and prevention.
Improving patient outcomes and population health can be achieved via timely intervention. Early illness detection allows healthcare practitioners to respond more quickly, resulting in improved treatment results and lower healthcare expenditures. By detecting illnesses at an early stage, healthcare professionals may adopt cost-effective treatments such as lifestyle changes or preventative drugs, which can be less expensive than treating the condition at an advanced level. Early detection can also assist to minimize costly hospitalizations and emergency visits.
The Role of Predictive Analytics in Early Disease Detection
By using complex algorithms and models to identify individuals who are at risk for certain conditions, predictive analysis is essential in early disease detection. By analyzing a wide variety of data, including clinical, demographic, and social determinants of health, predictive analytics may give significant insights into an individual’s health condition and potential future health concerns.
For example, predictive analytics can identify individuals who are at a higher risk of acquiring disorders such as diabetes, cardiovascular disease, or certain forms of cancer by analysing a person’s medical history, lifestyle choices, genetic information, and environmental variables.
Leveraging Predictive Models for Preventive Strategies
Employing predictive models for supporting the development of proactive healthcare treatments has substantial advantages.
By analysing numerous data sources such as medical records, lifestyle variables, and genetic information, healthcare practitioners can identify individuals who are at high risk of getting particular diseases. This allows them to undertake focused preventative strategies and interventions and put personalized care plans in place tailored to each individual. Individuals’ health outcomes, healthcare expenses, and quality of life can all benefit from this proactive approach.
One of the most significant advantages of predictive analytics is the capacity to detect high-risk individuals who do not yet display symptoms. By identifying these people early, healthcare practitioners can assist with preventative interventions like lifestyle changes and frequent checkups. This preventive strategy has the potential to considerably reduce disease burden and healthcare costs.
Improving Population Health Outcomes with Predictive Intelligence
Predictive analytics has the ability to alter healthcare’s focus from reactive to proactive, allowing healthcare organizations to better allocate resources and prioritize preventative interventions.
Between 1960 and 2022, healthcare spending in the United States increased from 5.0 to 17.9 percent of GDP (or USD 3.5 trillion), with an average increase of USD 146 to USD 10,739 per person!
Identifying high-risk patients and risk factors enables medical professionals to recommend preventative care strategies to lessen the likelihood of chronic disease development. ML-based algorithms have the potential to simultaneously employ complicated, non-linear correlations among several input risk predictors in contrast to traditional statistical risk prediction methods.
Overcoming Challenges and Ethical Considerations
Implementing predictive analytics for early illness identification and prevention presents its own set of obstacles. One key problem is resolving ethical concerns about data privacy, permission, and justice in predictive modeling.
It is critical to guarantee that individuals’ personal health data is secured and handled properly. In addition, fairness in predictive modeling should be enforced to minimize prejudice and discrimination. Striking a balance between using the potential of predictive analytics and adhering to ethical guidelines is critical for effective adoption in healthcare settings.
Future Directions and Advancements
Assessing the potential of artificial intelligence, machine learning, and big data analytics for early illness diagnosis and prevention entails investigating new trends in predictive analytics. These breakthroughs have the potential to improve prediction models by analyzing massive volumes of data, discovering trends, and forecasting illness risks.
Moreover, AI and machine learning systems may learn and improve over time, allowing for more accurate predictions. Big data analytics enables the integration of disparate data sources, resulting in a full picture of an individual’s health. These developments have the potential to transform early illness identification and preventive techniques.
Conclusion
This much has become clear: predictive analytics is critical in population health management for early illness identification and prevention. AI-based predictive models play a vital role in this early-stage identification by processing huge amounts of patient data like EHRs (Electronic Health Records), Patient-Generated Health Data (PGHD), lab test results, and more to identifying patterns and risk factors. Early identification allows providers to launch targeted interventions like screenings and medication, leading to mitigation, reduced ER admissions, or complete prevention in the best-case scenario. Such efforts not only improve patient outcomes but also translate to significant cost savings through value-based care.
Predictive analytics provides prompt interventions and personalized healthcare plans by harnessing modern technology and data analysis to improve treatment results and lower healthcare costs. To learn more about this topic, write to us at hello@felixsolutions.ai.