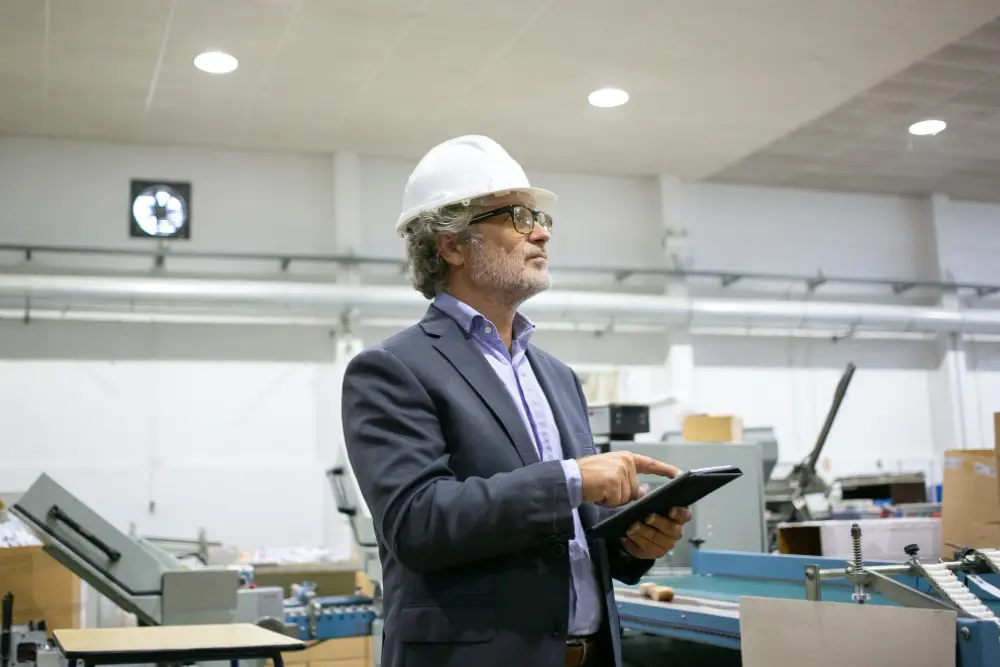
Businesses succeed when their products are loved by their users. However, building a product that users genuinely value is a lot more complex than it seems. Step one is gaining a deep understanding of user needs. Successful product engineering hinges on translating these needs into precise, actionable product specifications. As per research by the PDMA (Product Development and Management Association), products that are built with a strong focus on user needs have a greater success rate than those that don’t.
In this article, we’ll learn why user analysis is critical for optimizing product development and improving customer satisfaction and how enterprises can make this approach their core strategy.
User Insights: The First Step to Building Products People Love
If a built product doesn’t meet user expectations, it’s a failure. The failure rates of new product development differ from industry to industry, ranging from 35%-49%. Did you know that about 45% of features in a software product are never used? That is why gathering and analyzing user requirements is the cornerstone of any successful project, acting as a bridge between an initial idea and a product that truly meets users’ needs. This process helps teams discover what users really want, capturing essential details that influence the design, development, and delivery of the product. Let’s break down the many ways it is so important:
1. Building with Purpose
A product built without understanding its target audience is like trying to fix something that isn’t broken. In other words, NPD can be a wasteful and unnecessary process without user analysis. According to a recent report by Forrester, 70% of project failures happen due to a business’s inability to either attract or retain users. Gathering user requirements helps companies learn key insights into what are the expectations and pain points of users. This understanding directs the development process, ensuring every feature is crafted to serve a genuine need, preventing rework and saving time and money.
2. Reducing Development Risks
It’s a well-known fact that implementing changes later in the development process can be much more costly. The Systems Sciences Institute at IBM reported that fixing an error found after a product release is 100 times more expensive than doing it during design! When engineering teams invest time in user research and incorporate user feedback throughout the design process, they minimize the risk of costly rework down the road. Detailed user insights help avoid common pitfalls like implementing irrelevant features or missing out on essential ones, thus reducing the likelihood of product failure.
3. Enhancing User Satisfaction
User-centred products often lead to higher engagement and satisfaction because they resonate with users. When a product aligns with user needs, the likelihood of adoption and long-term retention increases, transforming users into advocates. In essence, products developed with a user focus become solutions users can’t live without. A quantitative study revealed that 22% of US online shoppers abandon an order due to long, complicated checkout processes. Research suggests that the average e-commerce site can improve its conversion rate by 35.26% just by making improvements to the checkout design alone. According to a PwC study, 73% of customers consider customer experience (CX) an important factor in their purchasing decisions, suggesting that products designed with a clear understanding of user needs lead to greater satisfaction and loyalty.
By knowing and prioritizing user requirements, enterprises can minimize costly revisions, avoid feature bloat, and deliver a solution that resonates with their audience. At last, this alignment reduces project risks, speeds up timelines, and leads to more user-centred results.
Designing with Goal: Translating User Insights into Product Features
Transforming user insights into product specifications is both an art and a science. By utilizing data-driven techniques, businesses can understand customer feedback and turn that into clear, prioritized requirements that guide product development. This process not only ensures that the final product resonates with users but also bridges the gap between customer needs and technical execution.
Among the manual methods, user interviews and surveys act as essential tools to capture user perspectives, each offering unique value. Interviews provide in-depth, qualitative insights into user expectations, while surveys gather large-scale trends and preferences, giving product teams invaluable data for defining core features. Adding to this, personas and user stories bring user-centric design to life. Personas can be defined as fictional yet data-driven profiles that represent the goals, pain points, and behaviours of target audience, keeping their needs at the front of development. Coupled with user stories, which outline tasks users aim to achieve, they provide engineers with a clear blueprint for creating relevant features. Finally, wireframes and prototypes play a crucial role in verifying these requirements early in the design process. By developing simplified versions of the product, designers allow users to interact and give feedback before full development, helping to uncover potential issues and saving time and resources.
Taking it a step further, AI techniques for translating user insights into product specifications provide a level of accuracy that manual methods often can’t match. By processing massive datasets, AI can detect patterns, sentiment shifts, and feature preferences at scale, uncovering trends that may be missed by human analysis. AI also reduces subjective biases, delivering objective insights rooted in data. This precision ensures that product specifications align closely with real user needs, accelerating development and enhancing the likelihood of product success.
Let’s explore key AI-based approaches that seamlessly convert user insights into impactful product features:
Natural Language Processing (NLP) for Sentiment and Topic Analysis: NLP enables the automated extraction of meaningful patterns and insights from huge amounts of user feedback like reviews, support tickets, and survey responses. Through sentiment analysis, teams can understand user pain points, preferences, and satisfaction levels, while topic modelling groups feedback by themes. Together, these techniques offer a structured view of user needs, enabling teams to prioritize feature development and align specifications with user expectations
Predictive Analytics for Feature Prioritization:
By leveraging predictive modelling, AI can determine how likely specific features are to enhance user engagement, retention, or satisfaction. To predict which features will have the most significant impact, these models look at factors like historical data, user personas, and market trends. Such data-driven approach allows design teams to prioritize features that are most likely to meet user needs and drive product success.
Machine Learning-Driven Clustering for Persona Creation:
Clustering algorithms in machine learning can categorize users based on similar behaviours, preferences, or demographics, enabling the creation of detailed personas. These personas help product teams understand diverse user needs and craft targeted specifications for each segment. Along with revealing hidden patterns in user data, clustering also allows for a more personalized approach to product development.
Customer Journey Mapping with AI:
User journey mapping visualizes the steps taken by a user to achieve a particular goal, helping teams identify opportunities for improvement within the user experience. By analyzing patterns in user interactions across platforms (web, mobile, social), AI-driven journey mapping tools can understand the emotional highs and lows along the user journey, and pinpoint where to focus feature development for maximum impact. As AI can identify nuanced user pathways and behaviours at scale which traditional techniques might miss, these insights guide UI/UX design improvements significantly.
Felix Solutions implements a user-centred approach to product development, tailoring its comprehensive AI/ML solutions to meet unique needs of the customer. From data preparation and feature engineering to model integration, Felix’s focus is on gaining maximum out of unstructured data through cutting-edge technologies like NLP. With a stage-based DevOps strategy, we align automation, configuration, and continuous delivery practices with business objectives, enabling consistent, high-quality software delivery. Through our platform engineering, cloud management, and enterprise architecture services, our team collaborates closely with clients to build scalable, sustainable solutions that drive innovation, agility, and growth.
Conclusion
Understanding user needs is the foundation of effective product engineering. By deeply engaging with users, product teams can transform raw data into clear, prioritized product specifications that drive success. Combining user research techniques with structured frameworks for prioritization enables businesses to deliver solutions that don’t just meet expectations but delight users at every turn.