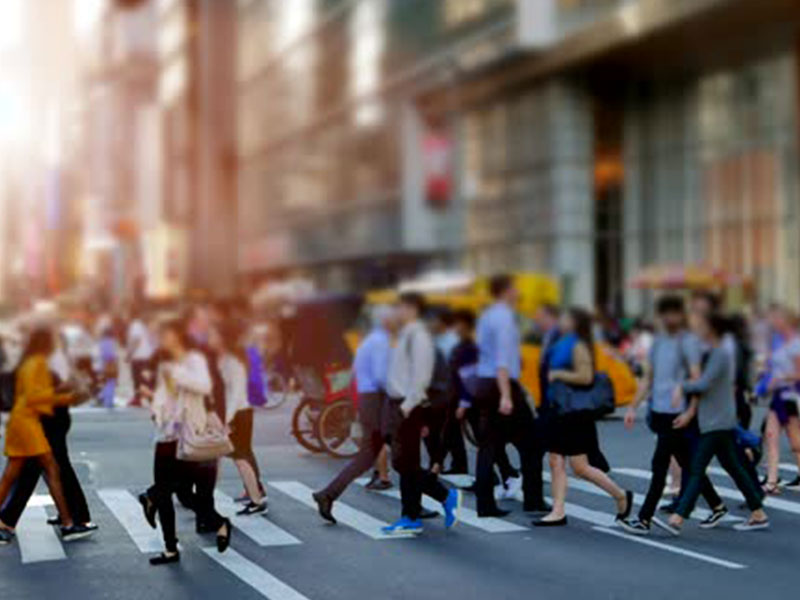
AI-driven Population Health Management (PHM) analytics can help healthcare providers identify patients who are at high risk for poor health outcomes and develop targeted interventions to engage and educate these patients. By working together with patients, healthcare providers can improve population health outcomes and promote better overall health for everyone.
AI-driven PHM analytics refers to the use of Artificial Intelligence (AI) technologies to analyze and manage population health data.This approach can help healthcare providers identify patterns and trends in patient health, predict potential health risks, and develop targeted interventions to enhance patient outcomes.
Limitatitons of Traditional Patient Engagement
Traditional patient engagement strategies, such as surveys and feedback mechanisms, have several limitations that can hinder their effectiveness in population health management.
First of all, these strategies often rely on self-reported data, which can be unreliable and incomplete. Patients may not always accurately report their symptoms, behaviors, or health status, resulting in inaccurate assessments of their health needs and ineffective interventions.
Secondly, traditional patient engagement strategies often lack personalization. Patients may receive generic messages or interventions that do not take into account their unique health needs, preferences, or circumstances, resulting in low engagement and poor outcomes.
Lastly, traditional patient engagement strategies may not be able to keep up with the pace of technological innovation. As new AI-driven technologies emerge, traditional strategies are becoming outdated and less effective.
The Role of AI-Driven PHM Analytics
To address these limitations, there is a need for a more data-driven and personalized approach to engaging patients in population health management. This approach can leverage AI-driven PHM analytics to analyze patient data in real-time, identify patterns, and develop tailored engagement strategies that meet each patient’s unique needs.
One specific AI-powered tool that can help providers analyze patient data is predictive analytics. This technology uses machine learning algorithms to analyze large amounts of patient data and identify patterns that can help predict future health outcomes. By using predictive analytics, providers can identify patients who are at risk of developing certain conditions and intervene early to prevent or manage them.
Natural language processing (NLP) is a powerful AI-tool that can be used by healthcare providers to better understand their patients. It can efficiently interpret patient feedback and point out the most frequent topics and moods. This information can be used to develop targeted engagement strategies that address patients’ concerns and improve their satisfaction.
Chatbots and virtual assistants are other examples of AI-powered tools that can help providers engage with their patients. These tools can be used to answer patient questions, provide personalized health recommendations, and even schedule appointments. Chatbots and virtual assistants are great tools that providers can use to enhance patient engagement while also significantly decreasing the workload of their personnel.
Use Case of AI-Driven PHM Analytics in Patient Engagement
Several healthcare organizations have successfully implemented AI-driven PHM analytics to improve patient understanding and engagement. For example, some are using AI-powered platforms to identify patients at risk of developing chronic conditions and provide them with personalized care plans. AI has brought a great many benefits to healthcare, including improved patient outcomes, fewer hospital visits and lower costs. Additionally, many organizations are now utilizing AI-powered chatbots to supply patients with tailored health information in order to further increase satisfaction and engagement rates while also bettering overall wellbeing.
Overcoming Barriers to Adoption
Implementing AI-driven PHM analytics in healthcare organizations can be challenging due to several potential barriers. One of the most significant concerns is data privacy. Healthcare organizations must ensure that patient data is protected and secure, and that any AI solution they implement complies with relevant HIPAA regulations.
To overcome this barrier, healthcare organizations can work with data privacy experts to develop robust data protection policies and procedures, and ensure that all staff members are properly trained on these policies. Another potential barrier is the need for interoperability between different healthcare systems and data sources. Healthcare organizations can invest in data integration solutions to aggregate data from other sources and systems.
Healthcare organizations can involve staff members in the implementation process and provide training and support to help them upskill and understand the benefits of AI solutions.
Conclusion
AI-driven PHM analytics have numerous advantages for healthcare organizations. It helps in better patient understanding and engagement, leading to higher patient satisfaction levels. Additionally, it can result in improved outcomes and reduced costs. It is essential to remember that effective population health management depends largely on patient understanding and engagement.
Patients who are knowledgeable and involved with their own care tend to stay on top of treatment plans, make healthier lifestyle changes, and ultimately experience better health results. This not only produces higher levels of patient satisfaction but also adds to the organization’s financial performance.
Additionally, AI-driven PHM analytics can help healthcare organizations reduce costs by optimizing care delivery and utilizing resources effectively. With the ever-increasing demand for better healthcare outcomes and cost-effectiveness, now is the time to explore and invest in AI-driven PHM analytics solutions.